Summary
Analysis
After a year and a half away from school to seek treatment for bipolar disorder, college student Kyle Behm had a friend recommend him for a job at a grocery store. But Kyle didn’t get an interview—and his friend later explained that Kyle had been “red-lighted” by a personality test he took as part of his application. Kyle applied to other jobs, and he was rejected from every single one. Kyle’s father, Roland, was an attorney. When he learned about what was going on, he sent notices to seven companies announcing his intent to file a class-action lawsuit, alleging that using the exam to weed out job applicants was unlawful.
Job-related personality tests are another form of WMDs that gather data about people and use that data in ways that are secretive or difficult to understand. They prioritize efficiency over fairness as they sort people into groups based on raw data alone. The job application process may be more streamlined in the present day compared to the past, but people aren’t necessarily being assessed fairly.
WMDs aren’t just corrupting the college admissions process or the criminal justice system—they’re hurting jobseekers, too. While looking for a job, people used to turn to their networks of friends and acquaintances for connections—so jobseekers who weren’t well-connected struggled to find work. Companies like Kronos that brought science into human resources in the 1970s promised to make the process fairer and eliminate some of “the guesswork” in hiring. But now that the hiring business brings in $500 million annually and employs tests and algorithms to weed out applicants, the job market is more unfair than ever before.
Personality tests are essentially used to exclude as many people from the hiring process as possible in order to make that process simpler and more streamlined. However, these tests sacrifice fairness for the sake of efficiency, because they ask leading and invasive questions that don’t necessarily determine what kind of worker or colleague a person would actually be. People’s applications—and indeed their lives—suffer as a result.
The problem with the use of these personality tests in the hiring process, O’Neil states, is that no one knows what the tests are looking for—the process is completely mysterious, the models are rarely updated or investigated to see how they’re excluding people, and they are increasingly common.
Because the methods and reasoning behind these personality tests are hidden, large-scale, and responsible for harmful feedback loops, they qualify as WMDs in O’Neil’s estimation.
Unsurprisingly, racial and ethnic minorities are most vulnerable to these application algorithms’ fallibilities. In the early 2000s, researchers from the University of Chicago and MIT send out 5,000 fake resumes for job openings at respected news outlets for a number of different roles. Each resume was modeled for race—half featured stereotypically “white” names, like Emily and Brendan, and others featured stereotypically “Black” names, like Jamaal and Lakisha. The “white” resumes got 50 percent more callbacks than the “Black” ones—and even “Black” resumes featuring strong qualifications were rejected outright. Even though it was automated, the hiring market was still “poisoned by prejudice.”
This anecdote illustrates how racial bias is often encoded in the job-hunting process. It furthers O’Neil’s argument that humanity needs to reassert its presence in some of these algorithms—many of which have gotten out of control over time. Encoded biases have begun to dictate outcomes like who doesn’t and doesn’t get hired at certain jobs, which results in racial discrimination.
Get the entire Weapons of Math Destruction LitChart as a printable PDF.
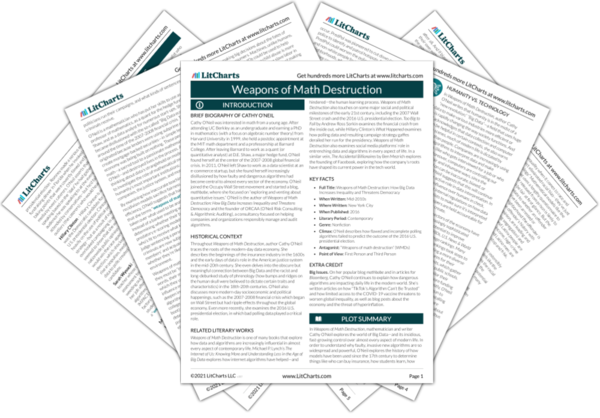
Human resources departments rely on these automated processes to help sift through huge numbers of resumes. Because these algorithms prioritize certain buzzwords and skills, they’re changing the way jobseekers write their resumes and cover letters. And those who have the money, time, and resources to prepare their materials based on insider information as to what the algorithms are looking for are those who wind up winning roles. At the same time, those from poor or disadvantaged communities often lose out.
The algorithms that now define the job-hunting process were meant to remedy human biases that can contaminate the process and create inequality. Yet now, these WMDs continue to treat people unequally—and they’re doing so on a larger scale than ever before.
In the 1970s, the admissions office at St. George’s Hospital Medical School in London needed a way to handle the massive number of applications they received each year. Administrators created a model that they believed would boost efficiency in culling applications while remaining fair and objective—but the very inputs that the humans taught the computer were biased and racist. The model they created excluded applicants with foreign names and rated female applicants lower in the system.
This passage illustrates how human bias can infiltrate even seemingly fair or objective algorithms. In this instance, the admissions model perpetuated racism and sexism—the opposite of what the admissions office set out to do.
In 1988, the British government found the school guilty of discrimination. Rather than axing female and immigrant applicants for whom childcare and language barriers might have been struggles, O’Neil suggests that St. George could have helped these worthy candidates and provided them with resources to make their careers easier and better. WMDs could help lots of people, but instead, they often serve unfair objectives.
Here, O’Neil illustrates how easy it would be for WMDs to work in the opposite direction—by singling out women and immigrants not to discriminate against them, but to elevate them and help them succeed. WMDs don’t have to be divisive and destructive. However, the humans that create and use these algorithms are often confirming or upholding their own biases, whether they intend to or not.
The objective of the WMDs created to filter out job candidates is almost always to reduce the risk of bad hires and cut down on administrative costs—in other words, they’re designed only to save money. These WMDs also try to filter out which candidates are most likely to stay at a job for a long time, preventing turnover (or “churn”) in the workplace. Those whose resumes reflect short stints at previous jobs or suggest that they’re a more “creative” or free-spirited type will score lower in some of these algorithms.
These WMDs are meant to efficiently and quickly find the most potentially reliable job candidates. But in the process of using data to sort people from one another, they punish applicants for their uniqueness and automatically reject potentially competent and talented prospective employees.
These WMDs took another dangerous thing into account: commute time. By removing access from applicants who live farther away from their jobs, these WMDs are directly contributing to feedback loops that keep poverty and immobility alive. Eventually, some companies did remove this metric from their models.
If a workplace is located in a high-income area, it’s usually the case that low-income employees will have to commute there. So, by ignoring candidates who lived far from their place of work, these algorithms were essentially telling low-income candidates that they weren’t worthy of the job. This perpetuates poverty, as it prevents those seeking upward mobility (higher income and class status) from reaching new opportunities.
Employers also tend to want to see whether someone will be a “team player” or not. Social networking sites like LinkedIn provide a glimpse into people’s social and work relationships. But Gild, a San Francisco-based start-up, sorts through millions of job sites to collect and analyze “social data,” attempting to quantify and qualify workers’ “social capital.” By tracking what sites people visit and what kinds of social media they engage with in their off-hours, Gild seeks to learn more about what kind of person an applicant is.
Even though Gild is trying to paint more holistic portraits of job applicants, their approach is problematic because busy workers who have families or offline pursuits and hobbies may not register as being sufficiently active and social online. Again, Gild’s algorithm is discriminatory, and it prioritizes efficiency over fairness.
Hiring models, O’Neil asserts, are prone to confirmation biases rooted in “pseudoscientific nonsense.” In this way, modern-day Big Data is a lot like phrenology—the racist and long-debunked study of whether irregularities of the human skull signaled things about personality and destiny. Like phrenology, a lot of the tenets that motivate Big Data are “untested assumptions.”
Even though the Big Data economy claims to offer solutions that will make life simpler and fairer, its methods aren’t vetted or regulated in any way. Thus, they have the potential to become harmful, widespread, and destructive—just like phrenology, a racist methodology that lumped certain groups of people together based on faulty logic.