One of the primary reasons behind the creation of algorithms that author Cathy O’Neil calls WMDs—“weapons of math destruction” that are widespread, harmful, and largely hidden from the public—was the desire to make various industries more equitable and efficient. In the late 20th and early 21st centuries, there was a sharp rise in the use of data to create mathematical models and algorithms that would help make schooling, credit scoring, and even criminal sentencing both easier and more just. But over time, in many cases, being efficient won out over being fair—and O’Neil suggests that today, the U.S. (and indeed the whole world) has been saddled with algorithms and systems that prioritize speed, ease, and profitability over fairness and equity. In order to reform WMDs, O’Neil asserts, data scientists and tech companies alike must begin sacrificing efficiency and profits for the sake of fairness, transparency, and morality.
Algorithms were initially created to be both more fair and more efficient than humans ever could be. To illustrate why mathematical models became important in restoring fairness to the economy, O’Neil offers a general example of a banker offering a newlywed couple in a small town a load in the 1960s. This banker might have conscious or unconscious biases against the couple. If he didn’t like their families, if they were a different race, or if he had some other prejudice against them, he could deny them a loan—even if they qualified for one. So, mathematical models were introduced to banking to remove these human biases from the equation. By quickly and efficiently determining who was creditworthy based on objective criteria, algorithms could create a fairer world.
Sixty years later, though, efficiency has won out over fairness—in O’Neil’s words, “the world is dominated by automatic systems.” O’Neil writes that “today, the success of a model is often measured in terms of profit [or] efficiency. But she questions whether these models are in fact actually “successful”—and whether society should redefine its idea of success. For example, advertisements that target internet users who are searching for information about food stamps sometimes collect information about these users, then use that information to target them with even more predatory ads—like ones promoting for-profit colleges. And recidivism models like the LSI-R are ostensibly efficient in determining which criminals are most likely to become repeat offenders. But by making huge determinations about people’s freedom based on data rather than a person’s humanity, these models also prioritize efficiency over fairness. When these algorithms rake in money or otherwise produce favorable results for companies and organizations, they’re considered a success—even though they take advantage of disenfranchised, vulnerable people in order to “succeed.” O’Neil suggests that when success is tied to efficiency and profit in this way, it means that fairness and equity aren’t part of the metrics of a successful algorithm. By preying on the poor and failing to prioritize justice and objectivity, algorithms are deepening inequality around the world.
Efficiency and profit, then, shouldn’t be the metrics of a successful model—fairness and equity should, and so companies must start to use their algorithms for good. There are models, O’Neil shows, that are already seeking to do good in the world. For example, Mira Bernstein, a Harvard PhD in mathematics, created a model that would scan industrial supply chains to look for signs of forced labor (or modern-day slavery) for a non-profit working to root out slave labor in the global economy. But models like this one, O’Neil states, are not prevalent enough. Algorithms and models are more frequently used to make the most profits in the most efficient way for big companies—models that prioritize fairness and that help nonprofits or other social justice initiatives reach their goals simply aren’t as valuable in the Big Data economy (the field that gathers and analyzes large sets of data). While algorithms were initially created to remove humanity from the equation and combine fairness and efficiency, the automatic nature of mathematical modeling has tilted toward efficiency over fairness. Now, O’Neil asserts, it’s time to put the humanity back into the picture rather than leaving the issue to the marketplace—which will, she predicts, always prize “efficiency, growth, and cash flow.”
Fairness vs. Efficiency ThemeTracker
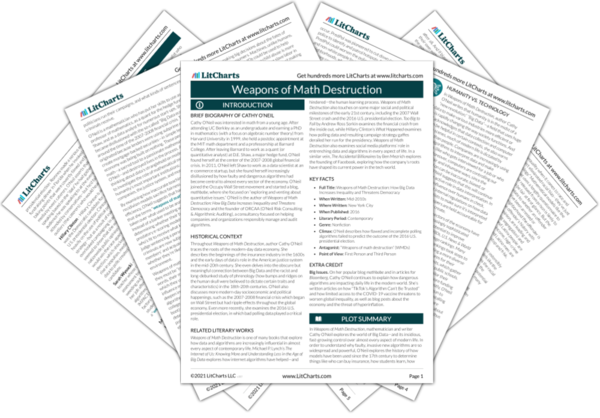
Fairness vs. Efficiency Quotes in Weapons of Math Destruction
The math-powered applications powering the data economy were based on choices made by fallible human beings. Some of these choices were no doubt made with the best intentions. Nevertheless, many of these models encoded human prejudice, misunderstanding, and bias into the software systems that increasingly managed our lives. Like gods, these mathematical models were opaque, their workings invisible to all but the highest priests in their domain: mathematicians and computer scientists. Their verdicts, even when wrong or harmful, were beyond dispute or appeal. And they tended to punish the poor and the oppressed in our society, while making the rich richer.
Do you see the paradox? An algorithm processes a slew of statistics and comes up with a probability that a certain person might be a bad hire, a risky borrower, a terrorist, or a miserable teacher. That probability is distilled into a score, which can turn someone’s life upside down. And yet when the person fights back, “suggestive” countervailing evidence simply won’t cut it. The case must be ironclad. The human victims of WMDs, we’ll see time and again, are held to a far higher standard of evidence than the algorithms themselves.
The value-added model in Washington, D.C., schools […] evaluates teachers largely on the basis of students’ test scores, while ignoring how much the teachers engage the students, work on specific skills, deal with classroom management, or help students with personal and family problems. It’s overly simple, sacrificing accuracy and insight for efficiency. Yet from the administrators’ perspective it provides an effective tool to ferret out hundreds of apparently underperforming teachers, even at the risk of misreading some of them.
And here’s one more thing about algorithms: they can leap from one field to the next, and they often do. Research in epidemiology can hold insights for box office predictions; spam filters are being retooled to identify the AIDS virus. This is true of WMDs as well. So if mathematical models in prisons appear to succeed at their job—which really boils down to efficient management of people—they could spread into the rest of the economy along with the other WMDs, leaving us as collateral damage.
That’s my point. This menace is rising.
What does a single national diet have to do with WMDs? Scale. A formula, whether it’s a diet or a tax code, might be perfectly innocuous in theory. But if it grows to become a national or global standard, it creates its own distorted and dystopian economy. This is what has happened in higher education.
It sounds like a joke, but they were absolutely serious. The stakes for the students were sky high. As they saw it, they faced a chance either to pursue an elite education and a prosperous career or to stay stuck in their provincial city, a relative backwater. And whether or not it was the case, they had the perception that others were cheating. So preventing the students in Zhongxiang from cheating was unfair. In a system in which cheating is the norm, following the rules amounts to a handicap.
The Internet provides advertisers with the greatest laboratory ever for consumer research and lead generation. […] Within hours […], each campaign can zero in on the most effective messages and come closer to reaching the glittering promise of all advertising: to reach a prospect at the right time, and with precisely the best message to trigger a decision, and thus succeed in hauling in another paying customer. This fine-tuning never stops.
And increasingly, the data-crunching machines are sifting through our data on their own, searching for our habits and hopes, fears and desires.
For-profit colleges, sadly, are hardly alone in deploying predatory ads. They have plenty of company. If you just think about where people are hurting, or desperate, you’ll find advertisers wielding their predatory models.
These types of low-level crimes populate their models with more and more dots, and the models send the cops back to the same neighborhood.
This creates a pernicious feedback loop. The policing itself spawns new data, which justifies more policing. And our prisons fill up with hundreds of thousands of people found guilty of victimless crimes. Most of them come from impoverished neighborhoods, and most are black or Hispanic. So even if a model is color blind, the result of it is anything but. In our largely segregated cities, geography is a highly effective proxy for race.
Police make choices about where they direct their attention. Today they focus almost exclusively on the poor. […] And now data scientists are stitching this status quo of the social order into models, like PredPol, that hold ever-greater sway over our lives.
The result is that while PredPol delivers a perfectly useful and even high-minded software tool, it is also a do-it-yourself WMD. In this sense, PredPol, even with the best of intentions, empowers police departments to zero in on the poor, stopping more of them, arresting a portion of those, and sending a subgroup to prison. […]
The result is that we criminalize poverty, believing all the while that our tools are not only scientific but fair.
While looking at WMDs, we’re often faced with a choice between fairness and efficacy. Our legal traditions lean strongly toward fairness. The Constitution, for example, presumes innocence and is engineered to value it. […]
WMDs, by contrast, tend to favor efficiency. By their very nature, they feed on data that can be measured and counted. But fairness is squishy and hard to quantify. It is a concept.
The hiring business is automating, and many of the new programs include personality tests like the one Kyle Behm took. It is now a $500 million annual business and is growing by 10 to 15 percent a year […]. Such tests now are used on 60 to 70 percent of prospective workers in the United States […].
Naturally, these hiring programs can't incorporate information about how the candidate would actually perform at the company. That’s in the future, and therefore unknown. So like many other Big Data programs, they settle for proxies. And as we’ve seen, proxies are bound to be inexact and often unfair.
The key is to analyze the skills each candidate brings […], not to fudge him or her by comparison with people who seem similar. What’s more, a bit of creative thinking at St. George’s could have addressed the challenges facing women and foreigners. […]
This is a point I’ll be returning to in future chapters: we’ve seen time and again that mathematical models can sift through data to locate people who are likely to face great challenges, whether from crime, poverty, or education. It’s up to society whether to use that intelligence to reject and punish them—or to reach out to them with the resources they need. We can use the scale and efficiency that make WMDs so pernicious in order to help people.
Phrenology was a model that relied on pseudoscientific nonsense to make authoritative pronouncements, and for decades it went untested. Big Data can fall into the same trap. Models like the ones that red-lighted Kyle Behm and blackballed foreign medical students at St. George’s can lock people out, even when the “science” inside them is little more than a bundle of untested assumptions.
With Big Data, […] businesses can now analyze customer traffic to calculate exactly how many employees they will need each hour of the day. The goal, of course, is to spend as little money as possible, which means keeping staffing at the bare minimum while making sure that reinforcements are on hand for the busy times.
But data studies that track employees’ behavior can also be used to cull a workforce. As the 2008 recession ripped through the economy, HR officials in the tech sector started to look at those Cataphora charts with a new purpose. They saw that some workers were represented as big dark circles, while others were smaller and dimmer. If they had to lay off workers, and most companies did, it made sense to start with the small and dim ones on the chart.
Were those workers really expendable? Again we come to digital phrenology. If a system designates a worker as a low idea generator or weak connector, that verdict becomes its own truth. That’s her score.
While its scores are meaningless, the impact of value-added modeling is pervasive and nefarious. “I’ve seen some great teachers convince themselves that they were mediocre at best based on those scores,” Clifford said. “It moved them away from the great lessons they used to teach, toward increasing test prep. To a young teacher, a poor value-added score is punishing, and a good one may lead to a false sense of accomplishment that has not been earned.”
Since [the invention of the FICO score], the use of scoring has of course proliferated wildly. Today we’re added up in every conceivable way as statisticians and mathematicians patch together a mishmash of data, from our zip codes and Internet surfing patterns to our recent purchases. Many of their pseudoscientific models attempt to predict our creditworthiness, giving each of us so-called e-scores. These numbers, which we rarely see, open doors for some of us, while slamming them in the face of others. Unlike the FICO scores they resemble, e-scores are arbitrary, unaccountable, unregulated, and often unfair—in short, they’re WMDs.
So why would [auto insurance companies’] models zero in on credit scores? Well, like other WMDs, automatic systems can plow through credit scores with great efficiency and at enormous scale. But I would argue that the chief reason has to do with profits.
But with such an immense laboratory for analytics at their fingertips, trucking companies aren’t stopping at safety. If you combine geoposition, onboard tracking technology, and cameras, truck drivers deliver a rich and constant stream of behavioral data. Trucking companies can now analyze different routes, assess fuel management, and compare results at different times of the day and night. They can even calculate ideal speeds for different road surfaces. And they use this data to figure out which patterns provide the most revenue at the lowest cost.
[Publicly held tech corporations’] profits are tightly linked to government policies. The government regulates them, or chooses not to, approves or blocks their mergers and acquisitions, and sets their tax policies (often turning a blind eye to the billions parked in offshore tax havens). This is why tech companies, like the rest of corporate America, inundate Washington with lobbyists and quietly pour hundreds of millions of dollars in contributions into the political system. Now they’re gaining the wherewithal to fine-tune our political behavior—and with it the shape of American government—just by tweaking their algorithms.
Big Data processes codify the past. They do not invent the future. Doing that requires moral imagination, and that's something only humans can provide. We have to explicitly embed better values into our algorithms, creating Big Data models that follow our ethical lead. Sometimes that will mean putting fairness ahead of profit.
Data is not going away. […] Predictive models are, increasingly, the tools we will be relying on to run our institutions, deploy our resources, and manage our lives. But as I’ve tried to show throughout this book, these models are constructed not just from data but from the choices we make about which data to pay attention to—and which to leave out. Those choices are not just about logistics, profits, and efficiency. They are fundamentally moral.
If we back away from them and treat mathematical models as a neutral and inevitable force […] we abdicate our responsibility. And the result, as we’ve seen, is WMDs that treat us like machine parts […] and feast on inequities. We must come together to police these WMDs, to tame and disarm them.