Big Data Quotes in Weapons of Math Destruction
The math-powered applications powering the data economy were based on choices made by fallible human beings. Some of these choices were no doubt made with the best intentions. Nevertheless, many of these models encoded human prejudice, misunderstanding, and bias into the software systems that increasingly managed our lives. Like gods, these mathematical models were opaque, their workings invisible to all but the highest priests in their domain: mathematicians and computer scientists. Their verdicts, even when wrong or harmful, were beyond dispute or appeal. And they tended to punish the poor and the oppressed in our society, while making the rich richer.
The hiring business is automating, and many of the new programs include personality tests like the one Kyle Behm took. It is now a $500 million annual business and is growing by 10 to 15 percent a year […]. Such tests now are used on 60 to 70 percent of prospective workers in the United States […].
Naturally, these hiring programs can't incorporate information about how the candidate would actually perform at the company. That’s in the future, and therefore unknown. So like many other Big Data programs, they settle for proxies. And as we’ve seen, proxies are bound to be inexact and often unfair.
Phrenology was a model that relied on pseudoscientific nonsense to make authoritative pronouncements, and for decades it went untested. Big Data can fall into the same trap. Models like the ones that red-lighted Kyle Behm and blackballed foreign medical students at St. George’s can lock people out, even when the “science” inside them is little more than a bundle of untested assumptions.
With Big Data, […] businesses can now analyze customer traffic to calculate exactly how many employees they will need each hour of the day. The goal, of course, is to spend as little money as possible, which means keeping staffing at the bare minimum while making sure that reinforcements are on hand for the busy times.
While its scores are meaningless, the impact of value-added modeling is pervasive and nefarious. “I’ve seen some great teachers convince themselves that they were mediocre at best based on those scores,” Clifford said. “It moved them away from the great lessons they used to teach, toward increasing test prep. To a young teacher, a poor value-added score is punishing, and a good one may lead to a false sense of accomplishment that has not been earned.”
Big Data processes codify the past. They do not invent the future. Doing that requires moral imagination, and that's something only humans can provide. We have to explicitly embed better values into our algorithms, creating Big Data models that follow our ethical lead. Sometimes that will mean putting fairness ahead of profit.
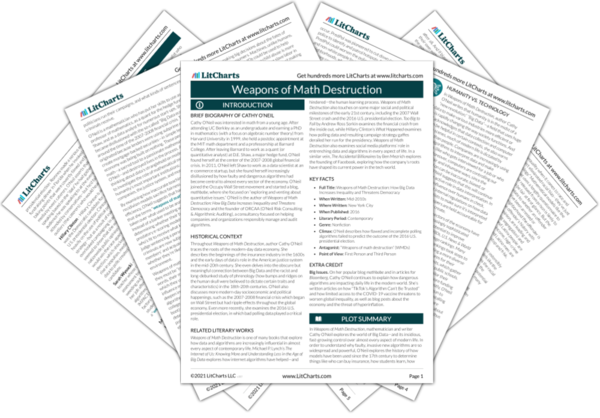