Summary
Analysis
In 1896, a German statistician named Frederick Hoffman who worked for the Prudential Life Insurance Company created a WMD. According to O’Neil, he published a 330-page report claiming that the lives of Black Americans were so precarious that “the entire race was uninsurable.” Like many other WMDs, Hoffman’s analysis was statistically flawed, racist, and unfortunately widespread.
WMDs, this passage illustrates, don’t necessarily need to be tied to sophisticated technology or complex algorithms. Any time that math is used in a way that’s difficult to understand and widely damaging, a WMD has been created.
For decades to come, insurers would cling to the idea that certain groups of people simply weren’t worth insuring. Bankers and insurance companies would start delineating neighborhoods that they wouldn’t invest in—this practice was called “redlining,” and it wasn’t outlawed until 1968. Yet redlining is still pervasive in U.S. society, and it’s coded into contemporary WMDs that use flawed statistics to punish poor people and racial or ethnic minorities.
The racist redlining of Black Americans was, no doubt, a WMD that created widespread harm and deepened social divisions in the U.S. Though redlining has been banned for several decades, it continues to echo throughout U.S. society in other forms.
Many WMDs that perpetuate redlining are found in the insurance sector. Insurance grew out of the predictive field of actuarial science. In the late 1600s, mathematicians discovered that by comparing mortality rates of different people within a given community, they could calculate probable arcs of people’s lives. Over the next several centuries, these predictions gave rise to the insurance business.
In the 1600s, math was used to create incredible predictions that had never been thought possible before. But in order to predict things like life expectancy, trends and similarities rather than individual circumstances became the metric by which people’s worth were measured. Individuals were lumped together for efficiency’s sake.
In today’s world, more data about people’s lives is available than ever before. Rather than making insurance predictions based on large groups, insurers are getting closer to being able to provide appropriate coverage based on the individual. Insurers use faulty proxies for responsible driving (like zip code and income) to create their own ratings, or e-scores—but because a lot of the information they use is based on credit and capital, insurance continues to work against the poor in many ways. Even drunk driving convictions count less in determining a person’s premium than credit scores. By ripping off desperate, working-class people, these companies make a fortune off good drivers with bad credit scores. And because the factors that go into pricing at major insurers like Allstate aren’t clear, their algorithms constitute WMDs.
Modern-day insurance continues to lump people together into certain categories using estimations and proxies rather than looking at an individual’s unique circumstances. This perpetuates social inequality by discriminating against low-income people who may be good drivers or homeowners but have poor credit. The WMDs used to determine who’s worthy of insurance aren’t fair by any means—they’re just efficient in terms of their ability to maximize insurers’ profits.
Get the entire Weapons of Math Destruction LitChart as a printable PDF.
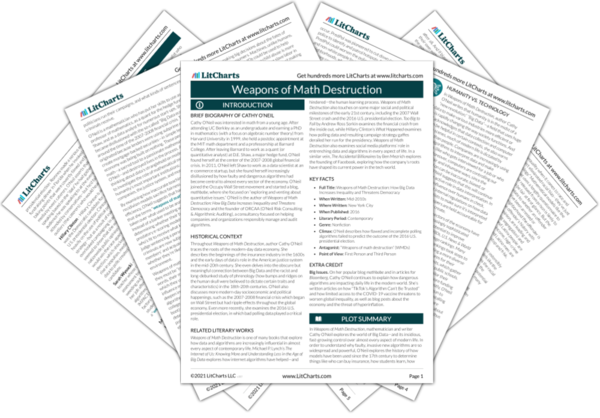
In the age of Big Data, insurers can judge us by how we drive in entirely new ways. In 2015, the U.S.’s largest trucking company (Swift Transportation) started installing cameras in long-haul trucks—one pointed at the road, the other at the driver’s face. The goal, according to Swift, was to reduce accidents—around 700 truckers die on the road in the U.S. each year. These fatal accidents are tragic, and they cost trucking companies a lot of insurance money (around $3.5 million per fatal crash). The additional surveillance also had another purpose, though: it let Swift gather a huge stream of data that could be used to optimize profits, compare individual drivers, and identify good performers.
Even though surveillance in truckers’ cabs was ostensibly being used to make trucking safer, its true purpose was to avoid costly payouts for the trucking corporations. This illustrates how WMDs are often touted as tools that will make life safer and fairer for working people—when in reality, they’re only used to maximize profits for companies. As such, this dynamic creates greater economic disparity.
Now, insurance companies offer regular drivers discounts if they agree to share their driving data through a small telemetric unit (like an airplane’s black box) placed inside the vehicle. This has the potential to help drivers save money—especially younger drivers, who are often costly to insure—but it’s also a big liability for poor or disadvantaged drivers. Driving through a bad neighborhood or providing evidence of a long commute each day might raise a driver’s rate. Eventually, the insurance companies’ promises to focus more on individuals becomes moot, because individual behavior is still being compared to that of others in similar demographics. While these systems are optional now, trackers, O’Neil asserts, will likely become the norm—and people will be punished for not having them rather than rewarded for consenting to them.
Again, O’Neil shows how, in the modern-day Big Data economy, more surveillance is often traded for a monetary break. So, working people who are desperate for insurance coverage, for instance, sacrifice their right to privacy in order to save money. This perpetuates economic disparity, and it sets a dangerous precedent for the future of surveillance and data-gathering techniques.
Insurance companies, O’Neil predicts, will soon start sorting people into new kinds of groups or “tribes” based on behavior. A decade ago, researchers at a data company called Sense Networks started to analyze cell phone data showing where people went. They could observe dots moving on maps to find similarities between groups of these dots. As the machine began sorting dots into different colors, only the machine knew what those colors meant—even Sense’s cofounder admitted that human observers wouldn’t be able to figure out what the “dots” had in common. This opacity, O’Neil asserts, is dangerous.
Major companies can now gather people’s data with complete impunity. They don’t even have to state what kind of data they’re gathering, or for what purpose they’re going to use that data. Data will continue to sort people, while most won’t know how or why they’re being sorted—in other words, humanity is now at the mercy of the assumptions that this advanced technology makes about them.
In 1943, because U.S. armies and industries needed every soldier or worker they could get, the Internal Revenue Service made a significant change: they gave tax-free status to employer-based health insurance. Within a decade, 65 percent of Americans were insured through their employers. This meant that employers gained a measure of control over their employees’ bodies. Today, employers can offer rewards or impose penalties through “wellness” programs. These programs can create initiatives like “HealthPoints” in which employees accrue “points” by taking a certain number of steps in a day or going for a check-up. In other words, companies can penalize workers who don’t consent to handing over data about their personal health.
Here, O’Neil shows how a measure taken in the name of efficiency—getting more people to enter the workforce at a crucial time—has slowly eroded privacy and allowed employers to get away with imposing judgment and bias on their employees.
Companies like Michelin have set employees goals for things like glucose, cholesterol, and waist size—employees who don’t reach goals in at least three categories (out of several) must pay extra toward their health insurance. And in 2013, CVS announced that if employees didn’t report their levels of body fat, blood sugar, blood pressure, and cholesterol, they’d have to pay $600 a year. This drew public ire, since the company used BMI (or body mass index) as a measurement of health—but BMI scores are “crude numerical prox[ies]” that were originally based around “average” male body types. In other words, their usefulness has been all but debunked.
The same way that nefarious e-scoring programs use proxy data like zip codes to determine who’s worthy of being insured, companies are now able to employ proxies like BMI to withhold or grant privileges to their employees. This widens inequality because BMI scores don’t necessarily give an accurate picture of a person’s health, so people with high BMIs may be unfairly penalized. In this way, employers essentially have permission to treat their employees unfairly based on outdated, flawed data.
Even though companies assert that they’re taking these invasive measures in the name of health, wellness programs don’t lead to lower healthcare spending—and there’s no evidence that they make workers healthier. O’Neil asserts that wellness programs aren’t yet full WMDs, since they’re often quite transparent. But they do show that employers are “overdosing” on employee data, trying to score potential workers and predict their productivity. If companies start creating their own health and productivity models, O’Neil suggests, the industry could very well become a full-fledged WMD.
Because these initiatives that pry into employees’ personal health information are geared toward providing employers with personal data about their workers, O’Neil implies that they’re classist and harmful. Without regulation, they could become the norm, and people’s personal freedoms will continue to erode.