Summary
Analysis
In previous decades, local bankers controlled the money in any given town. People would suit up and pay a visit to their banker if they needed a new car, a mortgage, or a loan. The bankers were people and neighbors—they knew about a person’s background and family in addition to having the numbers on their application form. In other words, the banker’s judgement was human and thus biased. For millions of people, the human angle of banking was a liability: if they were poor, Black, or female, they might have trouble convincing a banker to give them a loan. When Earl Isaac and Bill Fair developed the FICO model to evaluate the risk of an individual defaulting on a loan, things seemed to be looking up—with an algorithm doing the work, there’d be no bias in the credit process.
Securing credit used to be a much more human process—and thus a much more biased one. Technology has evolved to make the process both fairer and more efficient. Because of FICO scoring, a person’s credit history speaks for itself—and for many low-income people, non-white people, and women, that’s an important step toward a more just world. But because of the tension between efficiency and fairness that O’Neil has described in previous chapters, it’s clear that even a more objective process isn’t necessarily a perfectly equitable one.
While FICO scores were relatively transparent, fair, and backed by consistently updated data, the use of scoring has changed significantly over the years. “E-scores” now aggregate everything from zip codes to internet behavior to purchase history to create arbitrary, unregulated, and unfair WMDs. Companies like Neustar and Capital One score credit-seekers lightning-fast using metrics like location and internet history to determine who’s a worthy borrower. These e-scores create destructive rather than data-backed feedback loops. It’s not clear what metrics they use to determine who will get a loan, and they’re becoming more and more popular. By prioritizing efficiency over justice and transparency, they’re becoming predatory and unfair.
E-scores are taking society several steps backward from the fairness and transparency of the FICO scoring system. They’re not looking at individuals; they’re rating people in relation to a “blizzard of proxies.” So, while e-scores don’t do things like withhold credit from a Black lender based on race, they use things like zip codes—which can quite often be indicators of a person’s race or class—to assess how similar people have behaved in the past, rather than how the person seeking credit has behaved in the past. There’s no feedback that corrects these e-scoring systems when they make an error and arbitrarily place someone in the wrong category. And because the inner workings of e-scoring systems are hidden, no one can examine how they work in order to challenge or improve them.
E-scores are claiming to make the market fairer by using data that’s more objective—but they perpetuate racism and classism all the same. The longer these scoring systems remain unregulated, the more they threaten to become the standard for lenders. And the more powerful and widespread they become, the more they’ll deepen social divides by excluding minority and working-class people based on faulty proxy information rather than verified data.
Over time, creditworthiness has become a stand-in for virtues like a good work ethic and dependability, while bad credit signals “sins” that have nothing to do with being able to pay bills. Human resource management software now screens potential hires based on their credit reports, creating dangerous poverty cycles and feedback loops. “Framing debt as a moral issue,” O’Neil suggests, is a huge mistake.
Hard data like credit scores are replacing the emotional intelligence and nuance that humans bring to hiring and other screening processes. In this way, technology is erasing humanity from the processes that define our lives, turning human errors and missteps into “moral issues” that stand in the way of efficiency.
Get the entire Weapons of Math Destruction LitChart as a printable PDF.
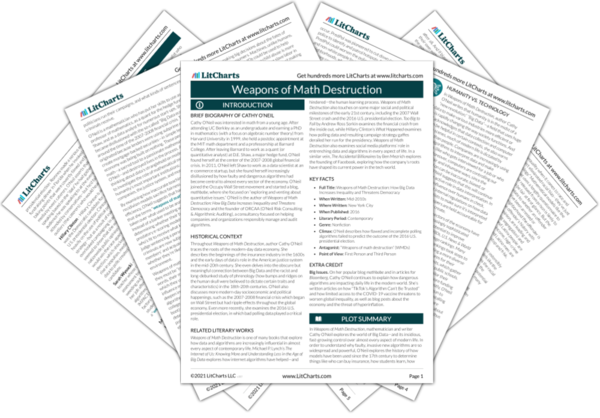
The systems that crunch numbers and run data about people’s lives aren’t perfect—no-fly lists are rife with errors that keep ethnic and religious minorities from traveling safely and easily, while wealthy white people can pay for “trusted traveler” status and bypass security altogether. Credit report errors can make borrowing difficult or impossible for people with great credit. And scoring algorithms often mix up common names, meaning that having the same name as someone with a criminal history or poor credit can be a liability.
Even though people are being told that any error or misstep is a huge liability, the systems that judge them are far from perfect. This double standard means that computers can get away with more than people can, setting a dangerous precedent for a future that’s increasingly governed by technology and data.
When an Arkansas woman named Catherine Taylor tried to secure federal housing assistance, she learned that her background check was full of mistakes and blended identities—she had many felonies on her report, some of which were tied to the alias of a woman named Chantel Taylor. Luckily, a housing authority employee helped Catherine find the errors and clean up the mess—but Catherine’s case is evidence of a larger problem with how these systems are built.
Catherine Taylor suffered consequences—a smeared reputation and trouble getting the federal assistance she was owed—due to faulty data. It took human beings to help right Catherine’s situation, whereas the technology that was judging her couldn’t be trusted.
As algorithms become more automatic, more errors pile up in people’s consumer profiles. These errors corrupt predictive models and give WMDs even more fuel. As computers become better able to learn from spoken language and images, these errors will only continue to pile up, creating uncountable instances of racist, unjust decisions made by faulty algorithms. These automatic systems now need a human hand to sift through their mistakes. Big Data needs to slow down and allow humans to play a greater role in sorting sensitive information, but the tech world is doubling down on predictive credit models.
This passage describes a dangerous domino effect that is already taking place within the tech world. Faulty data creates more faulty data—but broken algorithms aren’t vetted or regulated intensely enough, so the systems become more and more error-ridden over time. Unless humanity takes on a greater role in checking these systems over and thoroughly inspecting them, they’ll soon control more and more about how we live.
Facebook has recently patented a new type of credit rating based on social networks. For example, a young, white college graduate who spent five years volunteering in Africa might come home with no credit—but his connections on Facebook are successful and monied, and so he’s able to get a loan. But a hardworking Black or Latino housecleaner from a poor neighborhood whose social networks might reflect “friends” who are unemployed or incarcerated will have a harder time securing financial help.
O’Neil illustrates how algorithms meant to make things fairer or level a socioeconomic playing field often end up encoding biases that already exist in society. They create racist, classist feedback loops that deepen social divides and keep the disadvantaged from equal access to certain opportunities.
Meanwhile, credit card companies like American Express have come under fire for revoking or lowering credit for customers who shopped at certain kinds of establishments. This plays into the idea that someone spending their money at Saks Fifth Avenue is more likely to pay off their card each month than someone frequenting Walmart.
By judging people based on their social networks or shopping habits, algorithms like the one described here make faulty associations. And when those associations lead to actions like denying someone a loan, a job, or a higher credit limit, they deepen social inequality.
Companies like ZestFinance, a start-up that calculates risk and offers payday loans at a discount, buy up data about their customers in order to inform how big of a loan they get, and what their interest rate will be. People are trading privacy for discounts—and if Big Data algorithms find something as minor as a spelling error in a mountain of data about an individual, it could affect their credit score.
WMDs have changed the economy in huge ways already—so much so that people are volunteering their private information in hopes of scoring a deal. Data is the foundation of the economy, so faulty or error-ridden data poses a major problem.
“Peer-to-peer” lenders like Lending Club, which hoped to become a “new kind of bank” when it launched in 2007, used a combination of credit reports and data to run their operations. These companies can analyze any data they choose to and develop their own e-scores and risk correlations without explaining the methodologies behind them. O’Neil suggests that compared to the systems in place today, the prejudiced loan officers and bankers of long ago don’t look nearly as bad as they used to. At least borrowers, she writes, could “appeal to [their] humanity.”
As e-scores grow in power, they’re becoming less transparent and more capable of creating discriminatory feedback loops. So, while people might have faced judgement in the past, they could at least know what was counting against them. In today’s Big Data economy, there’s no transparency and no regulation, so it’s har for people to understand how to navigate this confusing new realm.