In the early pages of Weapons of Math Destruction, data scientist and author Cathy O’Neil shares her experience working at a major hedge fund at the onset of the 2007-2008 global financial crisis. At this time, she began to feel troubled when she realized that human bias is written into the algorithms that are used to determine crucial things like job proficiency, recidivism rates for criminals, creditworthiness, and insurability—especially because algorithms are supposed to be fairer and more objective than human beings. These harmful algorithms (which O’Neil calls “weapons of math destruction,” or WMDs) create unfair discrimination against women, racial and ethnic minorities, and low-income people. And O’Neil argues that unless developers, data scientists, and corporations actively work to purge sexism, racism, and classism from their algorithms, then women, racial minorities, and financially insecure people will be further victimized as time goes on.
WMDs create greater inequality when it comes to race and ethnicity. Algorithms haven’t eliminated racial bias—they’ve just “camouflaged it with technology.” Mathematical models like the LSI-R—a recidivism model that uses a lengthy questionnaire to determine whether an offender released from prison is likely to commit another crime—seem to be fair at first glance. But the questions it asks to gather data relate to whether an offender has friends or family members who’ve been arrested or incarcerated before, what kind of neighborhood the offender is planning on returning to, and what the offender’s history with police is. These questions, in O’Neil’s opinion, are leading and unfair. Due to redlining (a form of housing segregation) and other racist historical precedents, Black and Latinx people are often statistically more likely to live in low-income, high-crime neighborhoods. And because young Black and Latino men are disproportionately targeted by programs like stop and frisk, they’re likelier to have a record of prior police involvement—even if they were innocent. So, these ostensibly fair models are actually encoded with racism. Models like the LSI-R penalize non-white people by failing to address the racial biases that underpin U.S. society, which only deepens the country’s racial divide. Computer algorithms have the potential to help remedy the U.S.’s history of racism—but instead, many algorithms further perpetuate racial bias.
WMDs also deepen divides along the line of sex, preventing women from having fair shots at the same opportunities given to men. In the 1970s, the admissions office at St. George’s Hospital Medical School in London used an algorithm to sort through the many applications they received for a limited number of positions. The algorithm promised to make the applications process fairer and more efficient. But in practice, it systematically rejected resumes from people whose names seemed to indicate that they were immigrants or racial minorities, as well as from women (who might become mothers, impacting their value as laborers). While this anecdote happened several decades ago, it is an example of how human biases often infiltrate even seemingly “objective” programs when the data used to train the programs is itself encoded with bias. When algorithms are trained to discriminate against a certain kind of individual, this can have devastating implications. Women and racial minorities have long had to fight to assert their value as workers, and algorithms like the one employed by St. George’s threatened to make things even harder for female and non-white employees. By training machines to ignore information related to race, gender, or other categories, developers could ensure that their algorithms aren’t discriminatory—but all too often, models reflect their makers’ implicit biases.
Class in the U.S., too, is both exploited and cemented by harmful WMDs. To explain how WMDs play a role in perpetuating classism, O’Neil gives a hypothetical example of a working-class person who wants a fair rate on car insurance. Insurance companies use algorithms to determine insurance rates—and the data that feeds these algorithms isn’t always directly related to what kind of driver a person is. Someone might be a safe and skilled driver, which would entitle them to a reasonable rate. But insurers also take into account things like living in a low-income neighborhood where drunk drivers or car-jackings might be more common can become an unfair liability. Furthermore, people who live in low-income neighborhoods often commute to higher-income ones for work—and more time on the road means greater liabilities. So, a low-income person may receive a higher insurance rate not because of their driving record, but because of factors like location and driving time that they have little control over. Shift-scheduling is another realm that’s been largely automated by models and algorithms—but these algorithms prize efficiency over fairness. For instance, while it might save time and money for the same employee to close a store one night and open it the next morning, this creates stress and sleep deprivation for the employee. This kind of automated scheduling prevents working-class people from setting aside family time and investing in things like education and recreation—so it keeps them from enrolling in night school or pursuing a hobby, deepening the class divide practically as well as emotionally.
Because WMDs are unfair to women, working-class people, and minorities, O’Neil argues, they must be dismantled—in other words, companies and organizations must identify where they’re being used and begin regulating them. Otherwise, society will only grow more stratified, with disadvantaged groups entrapped by the very systems that claim to offer them a more equal social standing.
Discrimination in Algorithms ThemeTracker
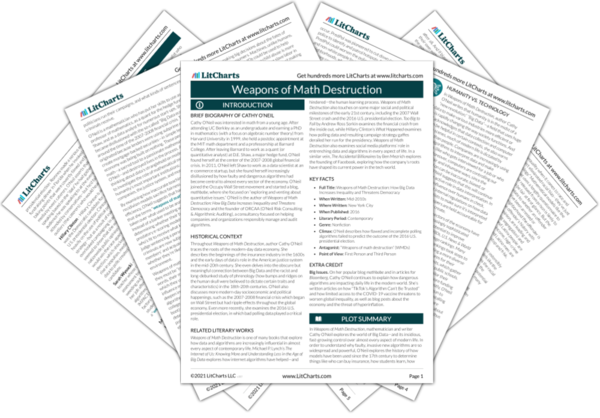
Discrimination in Algorithms Quotes in Weapons of Math Destruction
The math-powered applications powering the data economy were based on choices made by fallible human beings. Some of these choices were no doubt made with the best intentions. Nevertheless, many of these models encoded human prejudice, misunderstanding, and bias into the software systems that increasingly managed our lives. Like gods, these mathematical models were opaque, their workings invisible to all but the highest priests in their domain: mathematicians and computer scientists. Their verdicts, even when wrong or harmful, were beyond dispute or appeal. And they tended to punish the poor and the oppressed in our society, while making the rich richer.
Do you see the paradox? An algorithm processes a slew of statistics and comes up with a probability that a certain person might be a bad hire, a risky borrower, a terrorist, or a miserable teacher. That probability is distilled into a score, which can turn someone’s life upside down. And yet when the person fights back, “suggestive” countervailing evidence simply won’t cut it. The case must be ironclad. The human victims of WMDs, we’ll see time and again, are held to a far higher standard of evidence than the algorithms themselves.
And here’s one more thing about algorithms: they can leap from one field to the next, and they often do. Research in epidemiology can hold insights for box office predictions; spam filters are being retooled to identify the AIDS virus. This is true of WMDs as well. So if mathematical models in prisons appear to succeed at their job—which really boils down to efficient management of people—they could spread into the rest of the economy along with the other WMDs, leaving us as collateral damage.
That’s my point. This menace is rising.
It sounds like a joke, but they were absolutely serious. The stakes for the students were sky high. As they saw it, they faced a chance either to pursue an elite education and a prosperous career or to stay stuck in their provincial city, a relative backwater. And whether or not it was the case, they had the perception that others were cheating. So preventing the students in Zhongxiang from cheating was unfair. In a system in which cheating is the norm, following the rules amounts to a handicap.
The Internet provides advertisers with the greatest laboratory ever for consumer research and lead generation. […] Within hours […], each campaign can zero in on the most effective messages and come closer to reaching the glittering promise of all advertising: to reach a prospect at the right time, and with precisely the best message to trigger a decision, and thus succeed in hauling in another paying customer. This fine-tuning never stops.
And increasingly, the data-crunching machines are sifting through our data on their own, searching for our habits and hopes, fears and desires.
For-profit colleges, sadly, are hardly alone in deploying predatory ads. They have plenty of company. If you just think about where people are hurting, or desperate, you’ll find advertisers wielding their predatory models.
These types of low-level crimes populate their models with more and more dots, and the models send the cops back to the same neighborhood.
This creates a pernicious feedback loop. The policing itself spawns new data, which justifies more policing. And our prisons fill up with hundreds of thousands of people found guilty of victimless crimes. Most of them come from impoverished neighborhoods, and most are black or Hispanic. So even if a model is color blind, the result of it is anything but. In our largely segregated cities, geography is a highly effective proxy for race.
Police make choices about where they direct their attention. Today they focus almost exclusively on the poor. […] And now data scientists are stitching this status quo of the social order into models, like PredPol, that hold ever-greater sway over our lives.
The result is that while PredPol delivers a perfectly useful and even high-minded software tool, it is also a do-it-yourself WMD. In this sense, PredPol, even with the best of intentions, empowers police departments to zero in on the poor, stopping more of them, arresting a portion of those, and sending a subgroup to prison. […]
The result is that we criminalize poverty, believing all the while that our tools are not only scientific but fair.
While looking at WMDs, we’re often faced with a choice between fairness and efficacy. Our legal traditions lean strongly toward fairness. The Constitution, for example, presumes innocence and is engineered to value it. […]
WMDs, by contrast, tend to favor efficiency. By their very nature, they feed on data that can be measured and counted. But fairness is squishy and hard to quantify. It is a concept.
The key is to analyze the skills each candidate brings […], not to fudge him or her by comparison with people who seem similar. What’s more, a bit of creative thinking at St. George’s could have addressed the challenges facing women and foreigners. […]
This is a point I’ll be returning to in future chapters: we’ve seen time and again that mathematical models can sift through data to locate people who are likely to face great challenges, whether from crime, poverty, or education. It’s up to society whether to use that intelligence to reject and punish them—or to reach out to them with the resources they need. We can use the scale and efficiency that make WMDs so pernicious in order to help people.
Phrenology was a model that relied on pseudoscientific nonsense to make authoritative pronouncements, and for decades it went untested. Big Data can fall into the same trap. Models like the ones that red-lighted Kyle Behm and blackballed foreign medical students at St. George’s can lock people out, even when the “science” inside them is little more than a bundle of untested assumptions.
With Big Data, […] businesses can now analyze customer traffic to calculate exactly how many employees they will need each hour of the day. The goal, of course, is to spend as little money as possible, which means keeping staffing at the bare minimum while making sure that reinforcements are on hand for the busy times.
But data studies that track employees’ behavior can also be used to cull a workforce. As the 2008 recession ripped through the economy, HR officials in the tech sector started to look at those Cataphora charts with a new purpose. They saw that some workers were represented as big dark circles, while others were smaller and dimmer. If they had to lay off workers, and most companies did, it made sense to start with the small and dim ones on the chart.
Were those workers really expendable? Again we come to digital phrenology. If a system designates a worker as a low idea generator or weak connector, that verdict becomes its own truth. That’s her score.
While its scores are meaningless, the impact of value-added modeling is pervasive and nefarious. “I’ve seen some great teachers convince themselves that they were mediocre at best based on those scores,” Clifford said. “It moved them away from the great lessons they used to teach, toward increasing test prep. To a young teacher, a poor value-added score is punishing, and a good one may lead to a false sense of accomplishment that has not been earned.”
Since [the invention of the FICO score], the use of scoring has of course proliferated wildly. Today we’re added up in every conceivable way as statisticians and mathematicians patch together a mishmash of data, from our zip codes and Internet surfing patterns to our recent purchases. Many of their pseudoscientific models attempt to predict our creditworthiness, giving each of us so-called e-scores. These numbers, which we rarely see, open doors for some of us, while slamming them in the face of others. Unlike the FICO scores they resemble, e-scores are arbitrary, unaccountable, unregulated, and often unfair—in short, they’re WMDs.
So why would [auto insurance companies’] models zero in on credit scores? Well, like other WMDs, automatic systems can plow through credit scores with great efficiency and at enormous scale. But I would argue that the chief reason has to do with profits.
[Publicly held tech corporations’] profits are tightly linked to government policies. The government regulates them, or chooses not to, approves or blocks their mergers and acquisitions, and sets their tax policies (often turning a blind eye to the billions parked in offshore tax havens). This is why tech companies, like the rest of corporate America, inundate Washington with lobbyists and quietly pour hundreds of millions of dollars in contributions into the political system. Now they’re gaining the wherewithal to fine-tune our political behavior—and with it the shape of American government—just by tweaking their algorithms.
Successful microtargeting, in part, explains why in 2015 more than 43 percent of Republicans, according to a survey, still believed the lie that President Obama is a Muslim. And 20 percent of Americans believed he was born outside the United States and, consequently, an illegitimate president. (Democrats may well spread their own disinformation in microtargeting, but nothing that has surfaced matches the scale of the anti-Obama campaigns.)
Data is not going away. […] Predictive models are, increasingly, the tools we will be relying on to run our institutions, deploy our resources, and manage our lives. But as I’ve tried to show throughout this book, these models are constructed not just from data but from the choices we make about which data to pay attention to—and which to leave out. Those choices are not just about logistics, profits, and efficiency. They are fundamentally moral.
If we back away from them and treat mathematical models as a neutral and inevitable force […] we abdicate our responsibility. And the result, as we’ve seen, is WMDs that treat us like machine parts […] and feast on inequities. We must come together to police these WMDs, to tame and disarm them.